Civil Maps
The only scalable High Definition Mapping and Localization solution
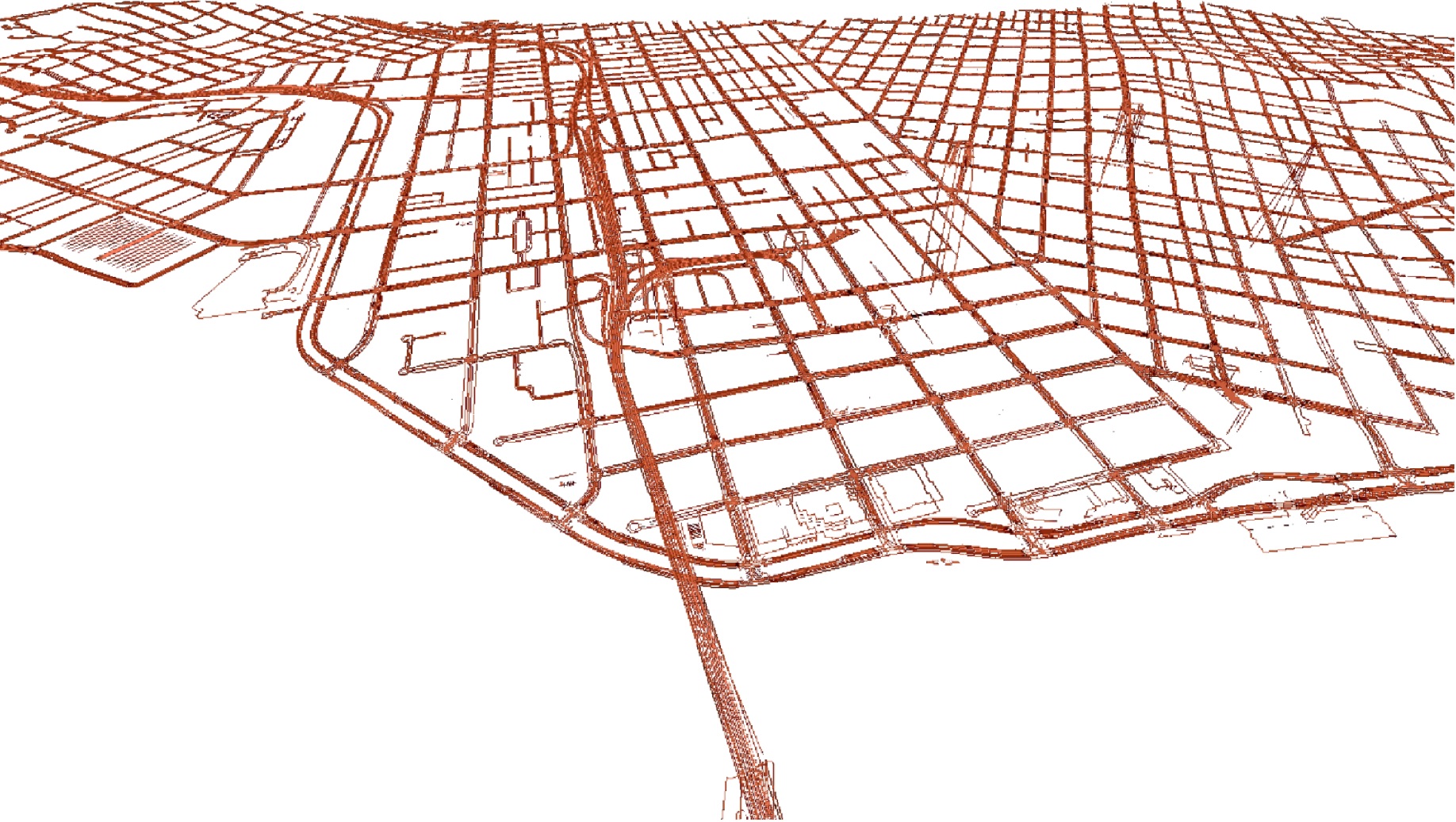
HD Mapping
Our highly scalable, patented and automated technology enables the delivery of HD Maps at city scale
- 15 cm – 20 cm absolute accuracy
- 1 cm – 5 cm relative accuracy
Berlin
Tokyo
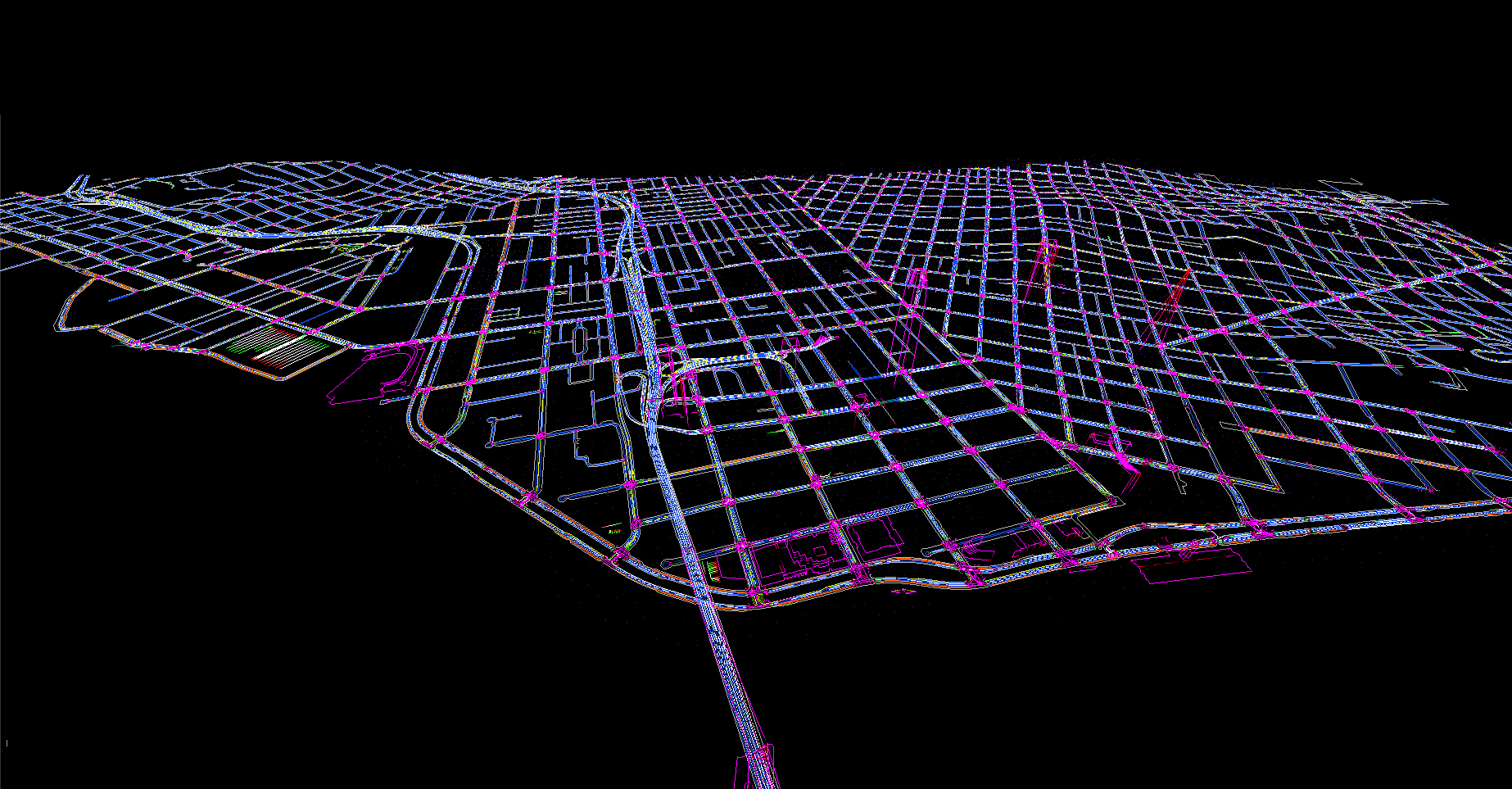
United States
San Francisco
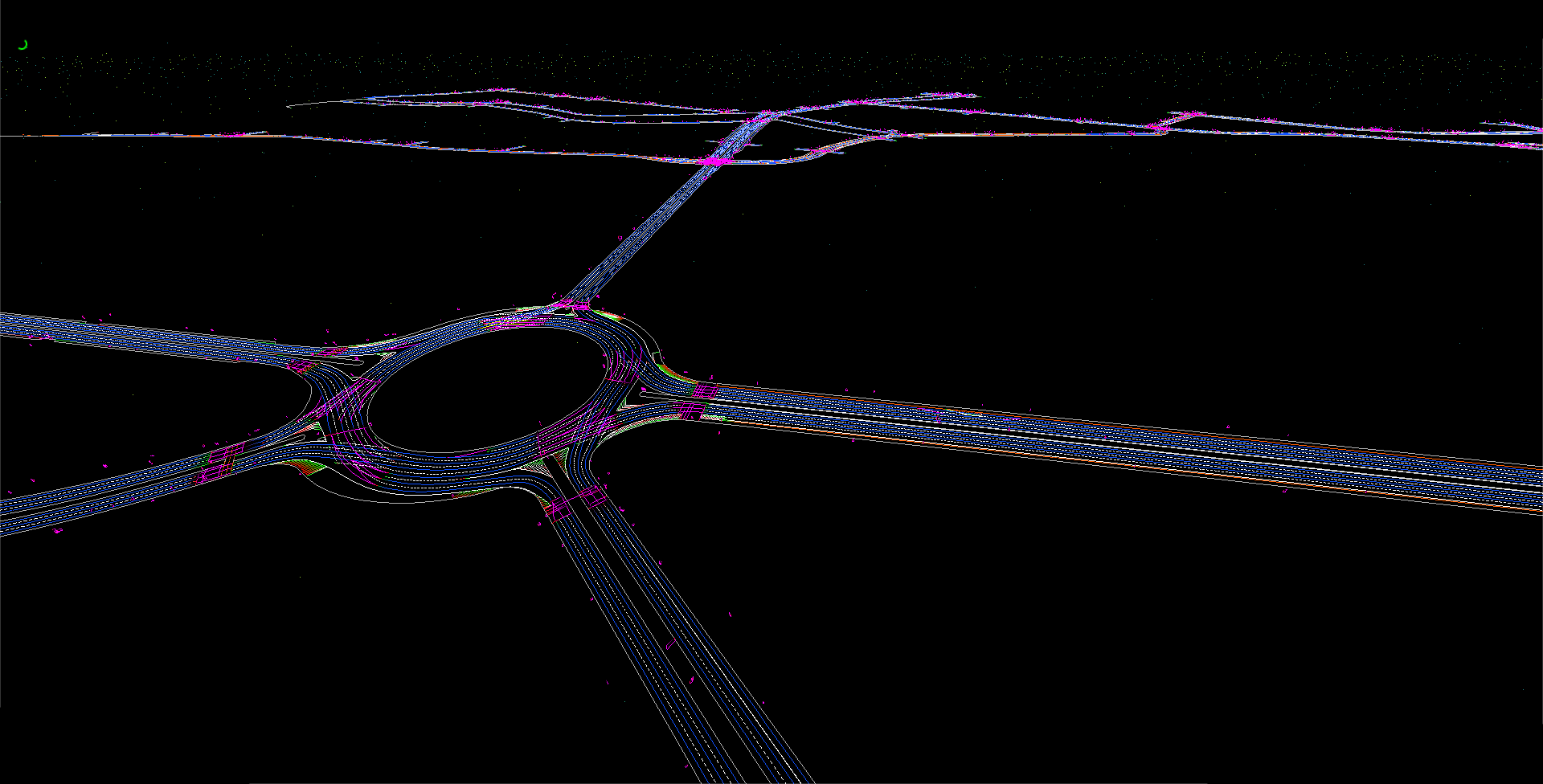
Europe
Berlin
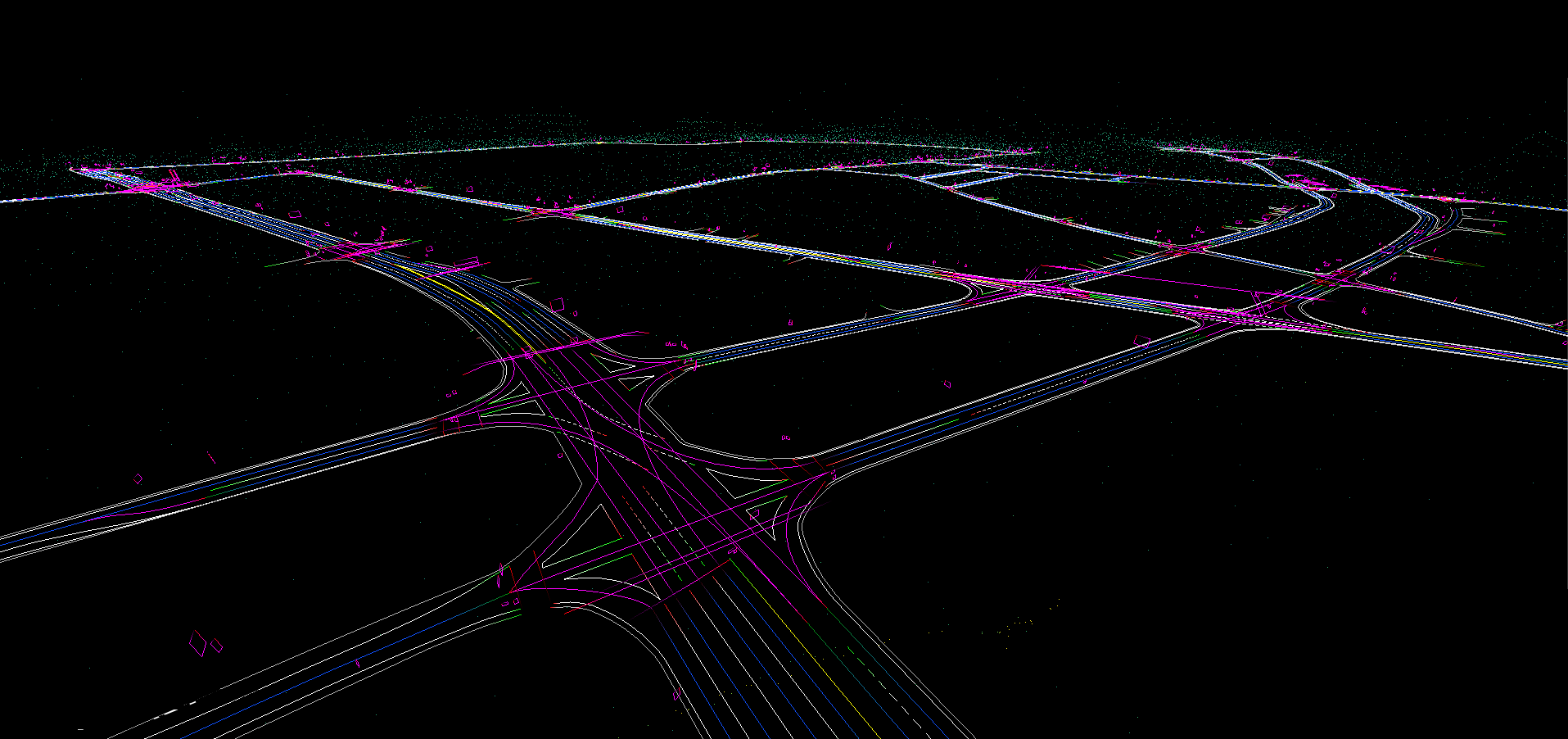
Asia
Tokyo
Localization
Civil Maps brings a unique, robust approach to real-time localization and navigation with our lightweight Fingerprint Base Map™.
This video was recorded live in San Francisco. Over 25 mins of continuous footage in dense, urban environment. Read more about our robust, lightweight localization product on our Company Blog.
Autonomous Vehicle Routing
Civil Maps AV Routing SDK powers the advanced navigational needs of autonomous vehicles, including sidewalk robots. Routing capabilities can be optimized to your business needs.
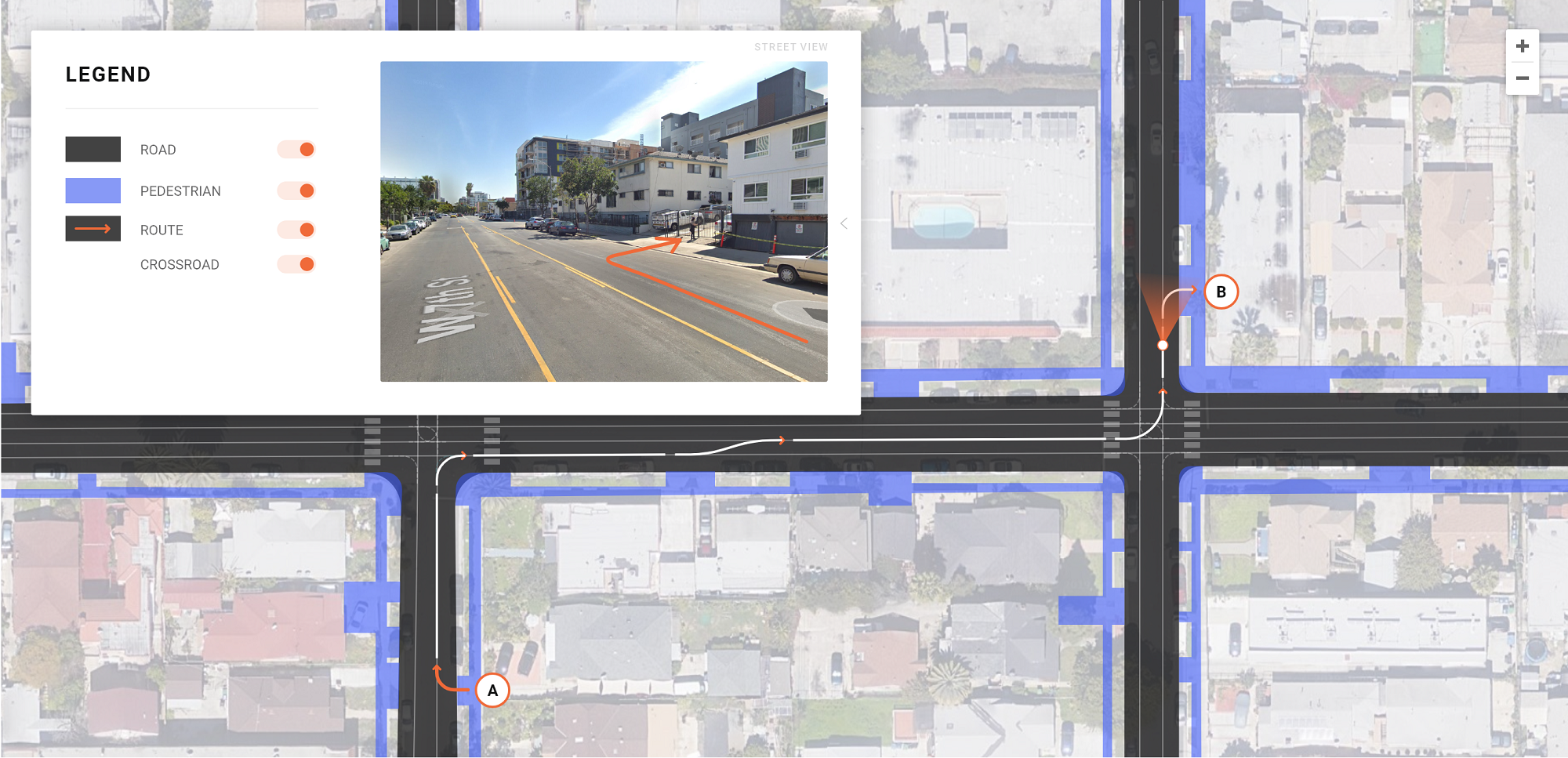
Our Team
We are a globally distributed team headquartered in San Francisco, with offices in Luxembourg, China, and India.
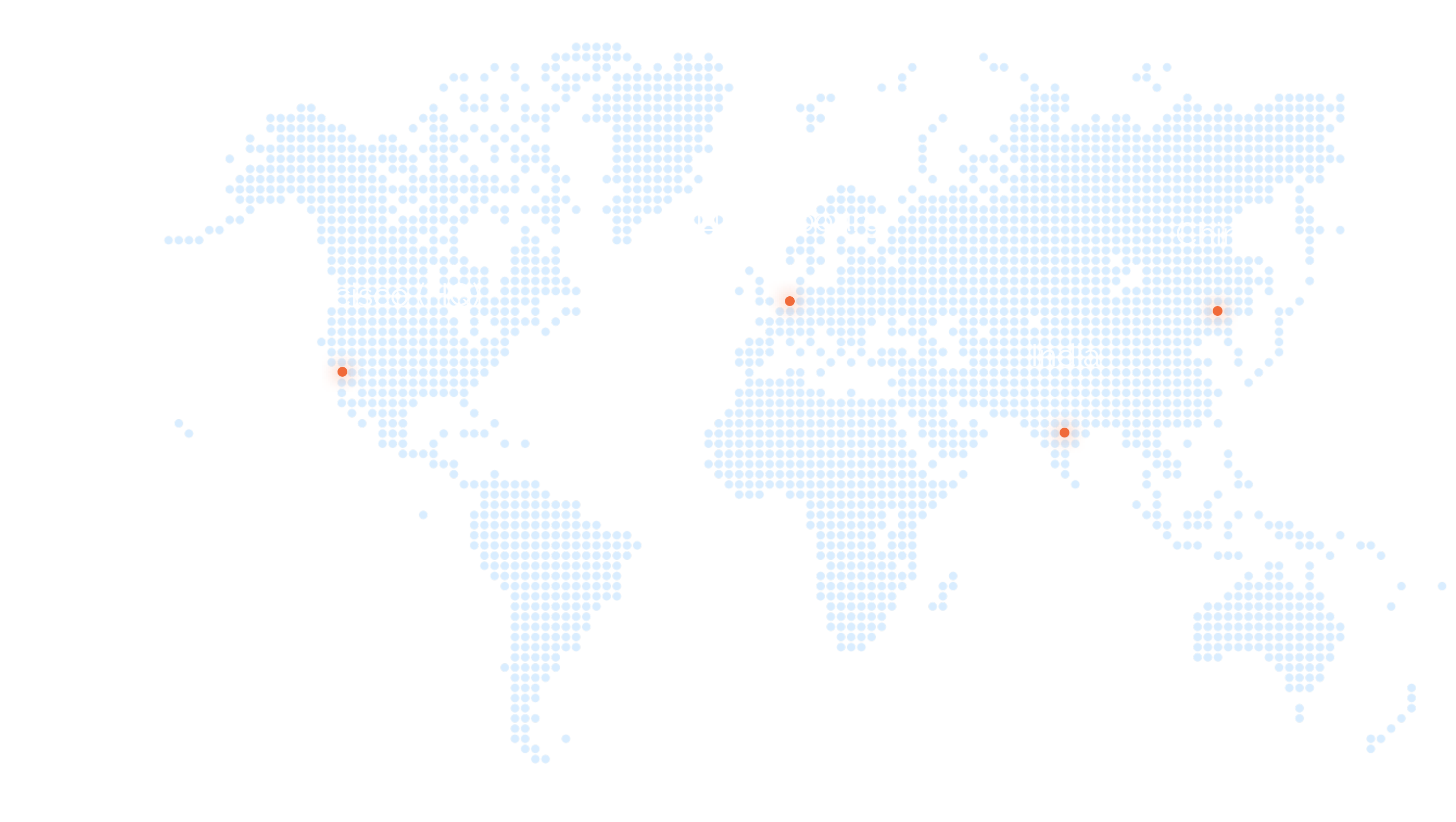